
Abstract
The capabilities of foundation models, most recently the Segment Anything Model, have gathered a large degree of attention for providing a versatile framework for tackling a wide array of image segmentation tasks. However, the interplay between human prompting strategies and the segmentation performance of these models remains understudied, as does the role played by the domain knowledge that humans (by previous exposure) and models (by pretraining) bring to the prompting process. To bridge this gap, we present the PointPrompt dataset compiled across multiple image modalities as well as multiple prompting annotators per modality. We collected a total of 16 image datasets from the natural, underwater, medical and seismic domain in order to create a comprehensive resource to facilitate the study of prompting behavior and agreement across modalities. Overall, our prompting dataset contains 158880 inclusion points and 52594 exclusion points over a total of 6000 images. Our analysis highlights the following: (i) viability of prompts across heterogeneous data, (ii) that point prompts are a valuable resource in the effort for enhancing the robustness and generalizability of segmentation models across diverse domains, (iii) prompts facilitate an understanding of the dynamics between annotation strategies and neural network outcomes.

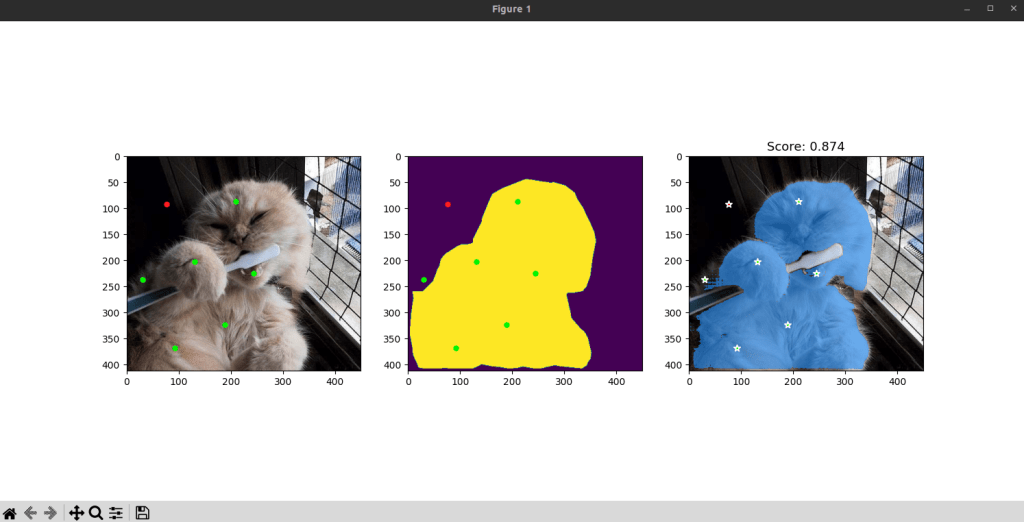
Dataset Download
Link: https://zenodo.org/records/10975868